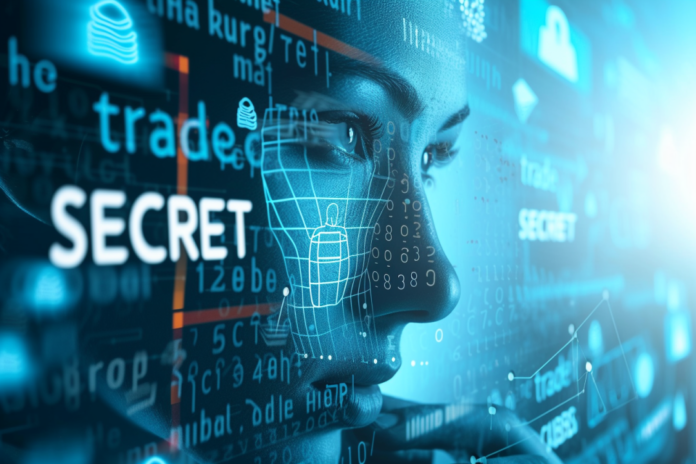
With generative AI technology advancing, the industry faces new challenges to the intellectual property system, from AI algorithm patentability and copyright implications of AI-assisted creations to AI’s role as a potential patent inventor and the handling of vast commercially valuable data generated by large models.
These developments heavily impact traditional IP protections. Trade secrets, a defensive IP right, bypass statutory authorization and the restrictive requirements of patents and copyrights, potentially offering a natural protection barrier for AI technology and applications.
Unique Trade Secret Challenges of Generative AI
Generative AI learns patterns from data to generate new outputs, using iterative training to improve accuracy. This process relies on algorithms and big data to conduct inductive reasoning, find rules, make predictions, and deliver results.
Data and algorithms are critical for accurate outputs, especially the weights generated during training, but they pose challenges for traditional copyright protection due to disputes over their status as copyrightable expressions. These elements, hidden in the model’s “black box,” align closely with trade secret confidentiality requirements.
In practice, users create new content by inputting prompts, which generative AI uses to predict results. If the input includes trade secrets, AI providers may inadvertently access these secrets. For example, Samsung faced a data breach when an employee used ChatGPT for work purposes, and Cyber Haven found that 11% of employee-pasted data into ChatGPT was confidential. Consequently, companies using generative AI are increasingly focused on protecting their trade secrets.
Given these issues, this article will analyze trade secret protection in AI research, development, and application from the perspectives of both AI technology providers and users.
Protecting Trade Secrets in AI Technology
Trade secrets are defined as technical, business, and commercial information not publicly known, holding commercial value and safeguarded by the right holder. To claim a trade secret, the right holder must show that the information is non-public, commercially valuable, and subject to reasonable confidentiality measures.
Generative AI often relies on data like public works, personal data, and trade secrets, assuming legal acquisition of training data. This article evaluates whether the data created through generative AI development, including model training and algorithm adjustments, qualifies for trade secret protection.
Generative AI development involves raw, labeled, and weighted data, mostly derived from public sources like books, academic papers, and media. While raw data is public and thus non-confidential, processed training data, weights, and other enterprise-handled data may qualify as trade secrets if confidentiality is maintained. However, trade secrets are vulnerable; any leakage undermines their value.
Moreover, right holders face challenges in defining the distinctive and non-public aspects and proving the information’s proprietary nature, particularly for large data sets where comparison is complex. To mitigate these vulnerabilities, data ownership rights may offer alternative protections.
Large AI models often build on open-source projects. Open-source code lacks trade secret protection due to public access, but modifications made by the enterprise may qualify if they remain confidential. However, certain open-source licenses require disclosure of derivative works, potentially complicating a company’s IP strategy. To avoid conflicts, R&D entities should review open-source license obligations carefully before using such models.
Algorithms, defined as rules for transforming input into output, may not qualify for copyright or patent protections, as they fall under intellectual activity methods. However, algorithm trade secrets face a unique challenge: transparency requirements. Regulatory frameworks like China’s Personal Information Protection Law, the EU’s AI Act, and the U.S. NIST’s Four Principles of Explainable AI require AI providers to explain their decision-making logic without full disclosure of proprietary details. China’s first algorithm trade secret case highlighted that even publicly known algorithms may be trade secrets if the enterprise has developed distinctive methods, such as unique settings and weights, that are commercially valuable and not publicly known.
Trade Secret Protection in AI Application Processes
When artificial intelligence processes user input containing trade secrets, it may generate content that builds on this input, potentially embodying trade secrets. Some argue that AI could even create trade secrets independently. Since AI service providers might access input data for model improvements, input content containing trade secrets risks exposure. Likewise, if output content with trade secrets is shared online, network security concerns may also pose a risk.
Despite these risks, AI’s efficiency gains make it essential to enterprise competitiveness, prompting businesses to pursue compliant, secure applications of AI. Enterprises should create comprehensive solutions addressing both input and output content handling, with special attention to personnel and systems involved in data flow. Determining if AI-generated content meets trade secret criteria is crucial.
In line with reasonable measures for trade secret protection, enterprises could adopt several strategies:
1. Using localized or internally deployed AI systems enables control over storage, processing, and generation within a secure, private cloud. Measures like data isolation, permission controls, and download restrictions enhance content confidentiality.
2. Guidance and training on compliant AI use are essential, especially as private deployment can be costly and limit real-time model updates. Training should cover trade secret scope, protection methods, risks, and benefits, detailing work content, desensitization, and storage practices as permitted.
3. Updating confidentiality clauses in procurement contracts ensures AI-specific terms are included. These should clarify input/output information’s confidentiality, ownership, and purpose restrictions, prohibiting use for model training, and define network security measures for storage, processing, and deletion.
4. Other technical measures, such as custom trade secret filtering tools, could be implemented to screen content before AI processing, offering an added layer of protection for enterprises with stringent confidentiality needs.
Source: Linkedin, Medium