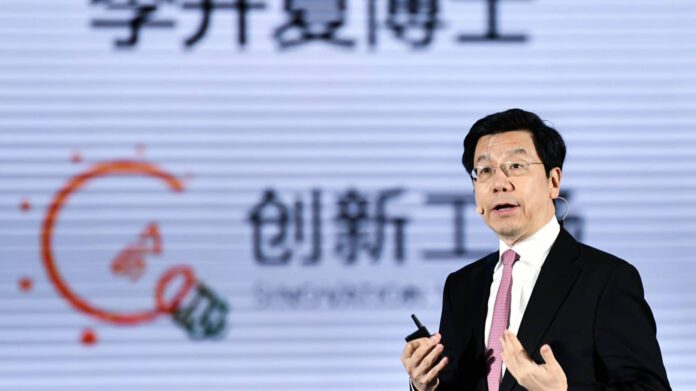
In May 2024, Lingyiwanwu launched Yi-Large, a model that quickly surpassed GPT-4 across various authoritative evaluation sets. By October, Yi-Lightning was introduced, exceeding the performance of GPT-4o, released by OpenAI in May, in the LMSYS Chatbot Arena, a well-known international blind test platform.
This remarkable progress indicates that Yi-Lightning managed to close the gap with the world’s leading model in a mere five to six months. However, accelerating this timeline further poses significant challenges for any Chinese AI company, particularly given the vast resources of global leaders like Google, Meta, OpenAI, and xAI, which have substantial advantages in terms of funding, computational power, and talent. At least for now, the resource-heavy approach employed by these companies is not easily replicable in China.
This raises an important question that the public have asked recently: should Chinese AI companies abandon pre-training models? This question reflects the growing anxiety within the industry, as some believe that the gap between Chinese models and global leaders is difficult to bridge.
Lee’s view is that the decision to abandon pre-training needs to consider three critical factors. First, whether the company has the financial resources to support pre-training. Many leading Chinese companies have raised enough funding to back such efforts, so this is not necessarily a prohibitive barrier. Second, whether the team is capable of executing pre-training effectively and achieving results that outperform existing open-source models. Chinese teams need to ensure that their models deliver significant advancements over current alternatives to justify the expense and effort. Finally, Chinese AI companies need to assess whether the return on investment for pre-training is worthwhile. This involves considering whether the trained model will have a long lifecycle and generate substantial commercial value.
While pre-training remains a valid approach for some companies, others may need to seek more pragmatic paths. Rather than attempting to replicate the resource-intensive model-building approaches of giants like OpenAI, Chinese companies should focus on areas where they can leverage their strengths—particularly in engineering and application implementation, where they excel globally.
Chinese AI teams are renowned for their ability to deliver exceptional engineering solutions, and they have proven themselves at the forefront of applied AI technology. Companies such as Lingyiwanwu have demonstrated how focusing on specialized areas can yield competitive advantages. Lingyiwanwu has built a world-class AI infrastructure team composed of top-tier international talent, enabling them to optimize their model training processes. By focusing on training efficiency, Lingyiwanwu has achieved a 99% effective training ratio, allowing them to build highly competitive models with fewer resources. This approach has allowed Yi-Lightning to be trained at a fraction of the cost of other leading models like Grok-2, yet it ranks alongside them on LMSYS.
While the ranking is impressive, Yi-Lightning’s true technological value is confirmed through authoritative reviews, showing that the model is not just competitive in terms of performance but also cost-effective. This strategy has led to Yi-Lightning being priced at just 0.99 RMB per million tokens, making it an attractive option for AI-native entrepreneurs looking for both high performance and low-cost solutions.
Yi-Lightning’s pre-training process cost approximately $3 million and involved 2000 graphics cards, but the model’s life cycle can be extended through post-training techniques. These include Supervised Fine-Tuning (SFT), Direct Preference Optimization (DPO), Online DPO, Proximal Policy Optimization (PPO), and inference, which together help extend the model’s usability for up to a year.
Extending the life cycle of models is critical for maximizing commercial returns and laying the groundwork for future model iterations. As the AI landscape evolves, focusing on these extensions of model utility will provide an ongoing advantage, allowing Chinese companies to refine and iterate upon their models with greater efficiency. Lingyiwanwu continues to explore further internal optimizations and will update its progress accordingly.
Beyond the technical aspects of model development, successful deployment is key to creating long-term value. Yi-Lightning, for example, is not just a high-performing model; it also has a highly competitive price point that makes it accessible to a wide range of users. This price-to-performance ratio ensures that it can drive commercial value by enabling businesses, particularly AI-native startups, to leverage the power of large models without incurring prohibitive costs.
Additionally, Lingyiwanwu is focusing on specific industry applications, such as retail solutions and smart computing centers, which have already garnered interest from major clients like Yum China. By focusing on specific application scenarios, Lingyiwanwu is positioning itself as a key player in the Chinese market, where the demand for AI solutions is growing rapidly.
Despite the clear resource disparity between Chinese companies and their Silicon Valley counterparts, there are areas where Chinese teams can still gain an edge. China boasts a large pool of skilled and diligent engineers who can drive more efficient model training processes, while its massive market and diverse application scenarios provide opportunities to deploy AI solutions at scale. By leveraging these strengths, Chinese companies can position themselves to lead the AI 2.0 era.
This era is defined not only by model development but by the ability to deploy and scale AI solutions quickly and cost-effectively. By focusing on building models that offer both performance and cost-efficiency, Chinese companies can promote the widespread adoption of large models, ultimately creating a self-reinforcing ecosystem that will fuel future innovation.
This strategy will allow Chinese AI companies to build a competitive advantage, overcoming resource limitations through smarter, more efficient development and deployment methods. It will also provide a solid foundation for the ongoing evolution of the AI industry in China, helping local companies eventually reach parity with, and potentially surpass, the current global leaders in the field. The combination of resource optimization, industry-specific solutions, and cost-effective model deployment offers a promising path for Chinese AI companies to lead the next wave of technological innovation.
Source: daxue consulting, zhihu, cnbc